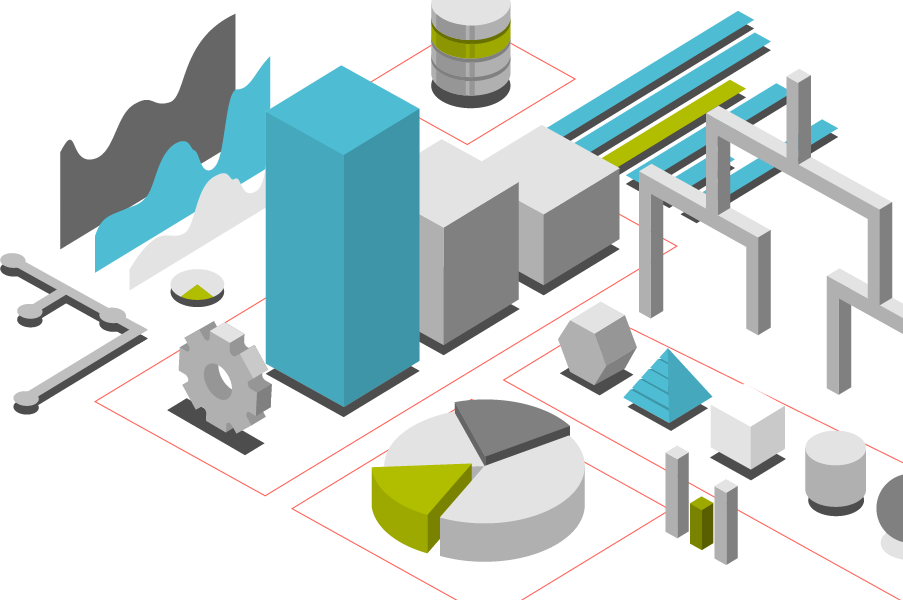
Content Management
Product Attributes
IDA helps clients establish the right attributes in PIM, and the right metadata and QA processes to enable effective maintenance over time. We focus on the attributes that drive user experience, product selection, AI, and revenue. Whether you are improving existing attribute data or building new data for PIM or ecommerce, schedule a demo today to se how we can use AI and data quality software get you there faster.
Product attributes are typically mastered within a “Master Attribution Hierarchy” in PIM, then sent downstream to websites, mobile apps, search applications, reporting environments and third parties. Attributes drive critical functionality like search results, search facets, selector attributes, product relationships and AI models.
​
Attributes are like an extension of the taxonomy. Designing the correct attributes for each category requires work due to the uniqueness of each catalog and the uniqueness of each PIM. If this is done well, so many other things can be automated.
​
The fact that attributes need to be consistent and complete is a given. The hard part is determining which attributes to maintain, finding scalable approaches to onboard and fill data, and right-sizing the approach to governance.
Product Descriptions
IDA helps clients leverage AI to automate as much of this work as possible while staying aligned with style guidelines and requirements.
Product descriptions reflect the personality of an organization and, along with images, serve as the “face” of the product. AI will do a lot of the work for you these days, but there are still style guidelines, character constraints, translations, trademarks, uniqueness and abbreviations to consider. Often times there are multiple descriptions for different contexts, each with its own set of requirements and guidelines.
Product Relationships
Relationships between products make catalogs easier to navigate and drive up average order values. Effective relationship management can require a blend of low-touch AI-generated relationships and high-touch relationships curated by subject matter experts.
​
There are several types of product relationships to consider -
-
Products Substitutes - Sometimes referred to as “functional equivalents,” these relationships allow users to identify intra-catalog alternate products. The identification of substitutes is often automated and rules based, with the quality of the output depending on the quality of the rules, prompts and QA. A subset of these substitutes may be identified as “recommended substitutes,” steering users towards preferred products based on quality, cost or profitability.
-
Compatible Products - These are products that are often used together, like a pipe and a pipe end cap. This requires the identification of products that should be used together vs. those that could be used together, and often require more planning and QA than other types of relationships.
-
Replacement Parts - These relationships link complex products, like equipment and machinery, to their replacement parts. These relationships help create equipment-specific user experiences, where a user can view available replacement parts.
-
Complementary Products - These relationships are typically the most loosely defined and only require that the items can be used together. Examples include hand tools and work gloves or cleaning chemicals and cleaning brushes.
Digital Assets
Whether you are sourcing new assets or rationalizing existing assets, IDA will help you build a complete and consistent asset library that gives you a competitive advantage, while automating as much of the process as possible.
Whether your DAM stands alone or is part of a PIM, IDA makes it easy to maintain digital assets and their relationships with SKUs, variable products, attributes and categories. Asset assignments, prioritization, duplicate identification, and metadata management has been revolutionized by AI.
Data Fill
Whether you are sourcing new assets or rationalizing existing assets, IDA will help you build a complete and consistent asset library that gives you a competitive advantage, while automating as much of the process as possible.
A catalog with 100,000 products will contain millions of publishable item-level attribute values. These values drive critical search functionalities, provide insights in reports, compare products, and answer millions of customer and employee questions all day long. Everybody knows that the data needs to be filled and it needs to be accurate, but finding the sweet spot between price and quality can be challenging.
​
Like item classification and some copywriting, data fill work has become an “AI first” activity. We use AI to mine descriptions, websites, data pools and pdfs before handing off to Data Analysts, who then use purpose-built QA tools to enrich and finalize data before publishing. In some cases, when source data is complex or highly inconsistent, we still use old-fashioned single-key and double-key data entry processes.
​
Data fill projects are all about metrics. There are two key metrics in an IDA data fill project -
-
Data accuracy estimate - We estimate the product information accuracy rate before and after the project.
-
Data fill rate - We report on before and after fill rates, including summaries by category, by attribute and by brand.
​
While AI has been a game-changer for B2B data fill, it has also created an epidemic of false promises and failed projects. The tough lesson - you can’t lock in AI cost savings by neglecting QA. IDA helps clients shift to lower cost data fill methodologies while increasing (not decreasing) data accuracy.